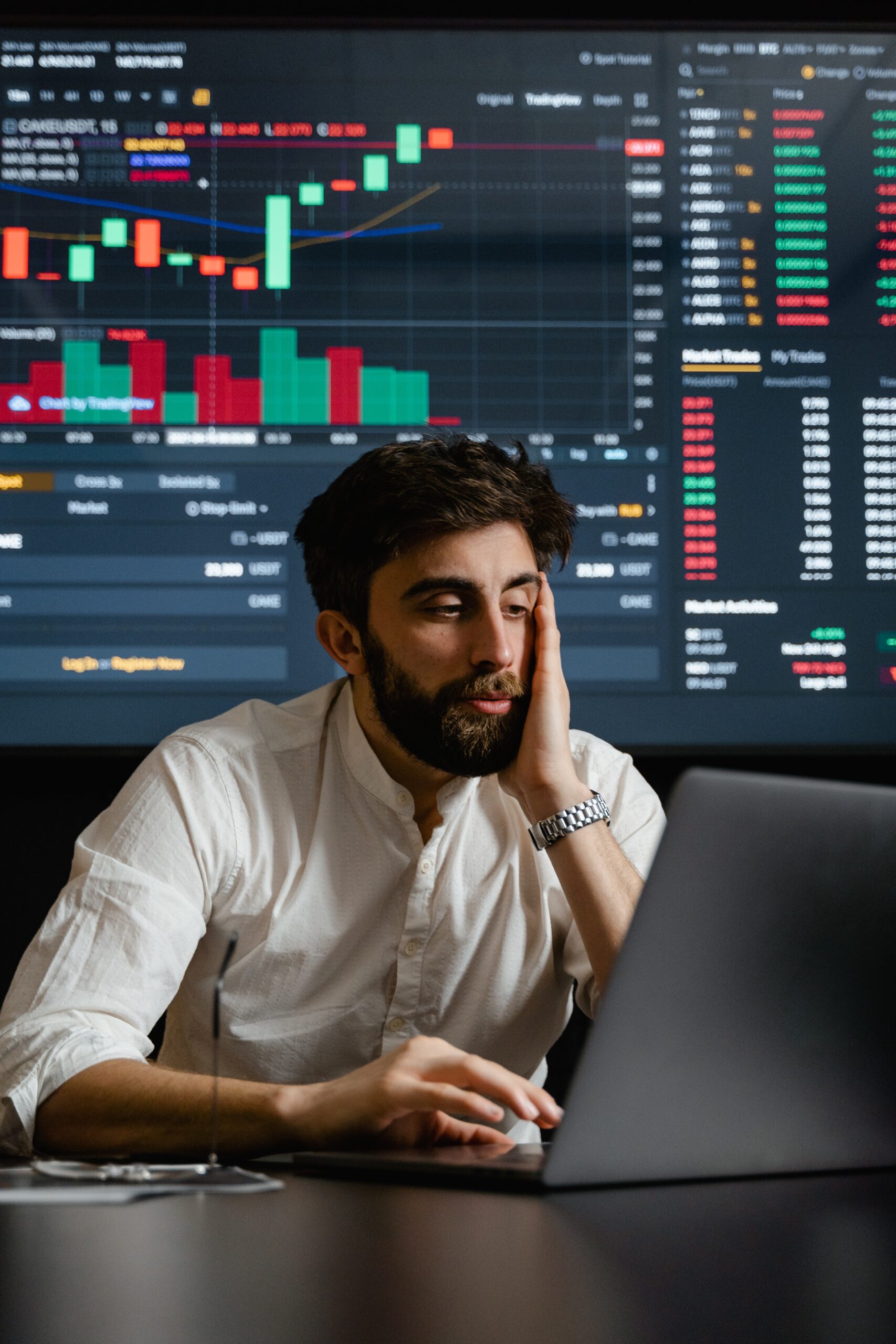
Comprehensive Location Intelligence Across the Globe
Why is International Location Data So Inaccurate?
As organizations around the globe attempt to keep up with consumer and business trends, more and more are turning to location data to stay ahead of the pack. Armed with demographic, mobility, and POI (point of interest) datasets, leaders from every industry are looking to develop intelligence about customers and competitors that will help them scale internationally.
It’s no secret that the global insights provided by accurate geospatial data are a valuable asset. Where demographic data can offer dynamic customer profiles, mobility data makes it possible to map buying and transportation habits as well as time spent in brick-and-mortars. For its part, POI data gives brands a holistic view not only of competitor locations but also of complementary or high-risk sites of interest capable of driving visits and sales (or not).
But there’s a problem: a huge percentage of international location data is inaccurate. Let’s zoom out to better understand the true costs of poor-quality data before considering a case study that illustrates what can go wrong with location data—as well as how to fix it.
Learning the cost of bad data
Imagine that a quick service restaurant (QSR) wants to use POI datasets to increase their international competitive intelligence. They know that this kind of data has the potential to increase distribution efficiency, inform strategic decision making about site selection, and allow them to reach new markets and customers.

What’s less obvious, however, is where the QSR should look for accurate datasets. POI data has numerous sources, ranging from state governments to private companies. Some of these provide their datasets for free, while others make them available for purchase either directly or through a location intelligence platform.
Presented with these options, it’s critical that the QSR (and every company using geospatial datasets, for that matter) follow best practices when selecting a data source. The stakes are high if they don’t: inaccurate data can result in lower customer retention as well as significant over- or under-investment. These losses of money and time could set company strategy back months (or even years) and risk eroding shareholder confidence.
Case study: Developing location intelligence in Mexico
Let’s get even more specific and imagine that Taco Bell wants to take another swing at setting up shop in Mexico, one of the world’s most open and attractive markets for international brands. After past difficulties, the QSR decides that this time will be different—in part because they’ve got international location data on their side.
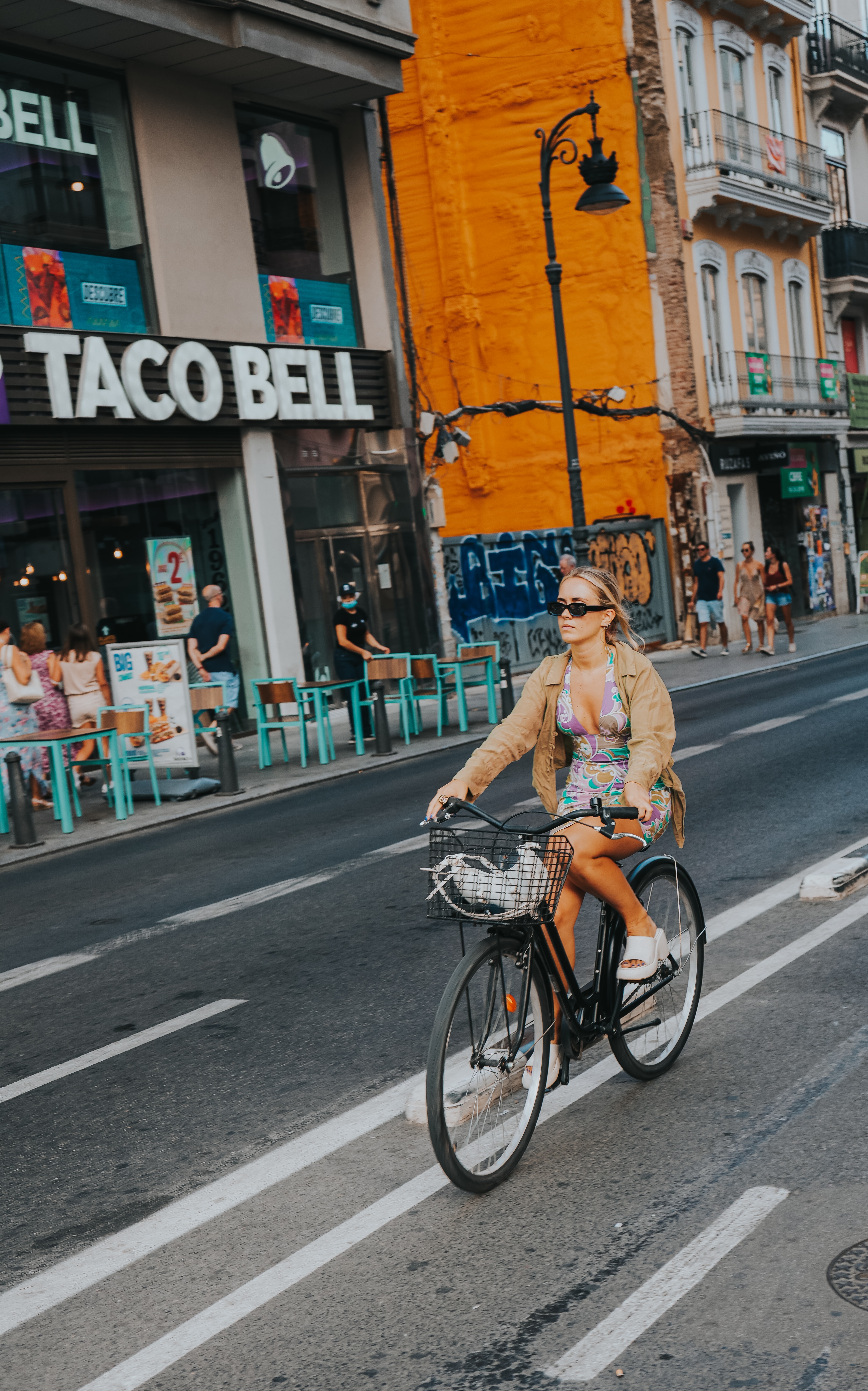
Taco Bell knows that they can develop insights on the basis of the four main attributes of POI records. These include location, function (or place type), contact information, and franchise information. They source this data for free from the Instituto Nacional de Estadística y Geografía (INEGI), which organizations the world over rely on for information about local businesses in Mexico.
With these data points in hand, Taco Bell plans to develop an insight-forward strategy to site selection in Cancún that avoids locations already rife with local dining options and maximizes proximity to complementary businesses.
But, again, there’s a problem.
A study by dataPlor shows that from a random sample of roughly 1,000 INEGI business records, 80% of the data about Mexico POIs is inaccurate. For starters, these records’ contact information is highly unreliable: in the sample, 50% of listed websites were incorrect, and 81% of phone numbers were missing or incorrect. Three percent of these records were stuck with the wrong address, making them functionally invisible to anyone working with INEGI’s dataset.
Often, international POI records are incorrect because they have been pulled from multiple sources. Left unverified, this data patchwork—whose points could come from erroneous in-person observations or out-of-date information from websites such as Google Maps or TripAdvisor—might never be corrected.
Whatever the reason for these inaccuracies, the study above highlights how unreliable international location data can be and points to the complications that can arise when sourcing it for free. Indeed, were Taco Bell to craft growth strategies on the basis of these datasets, a lot would go wrong. In Cancún, for instance, 91% of the INEGI records sampled contained some form of inaccuracy. Making decisions with this data would likely cause the company to misunderstand their competition, set up shop in suboptimal locations, and end up courting the wrong audiences. For example, they might fail to see an after-hours market opportunity in a bustling downtown neighborhood or might otherwise open a store on a street full of popular mom-and-pop options.
In order to avoid mistakes like these, organizations looking to win internationally need to scrutinize the sources of their location data. When vetting a source, it’s critical to make sure that 1) they in fact specialize in geospatial data, 2) they collate multiple inputs for each record, 3) they offer metadata and other indicators that ensure records’ accuracy, and 4) they rely on human sources for reliable, in-person verification. Also, it’s important to consider whether they enhance their data to ensure its accuracy—something from which INEGI’s datasets would surely benefit. By following these simple steps, companies can harness the power of location data and become truly global leaders.