Comprehensive Location Intelligence Across the Globe
Grow your business with confidence, backed by the most accurate and globally scaled location data in the industry.
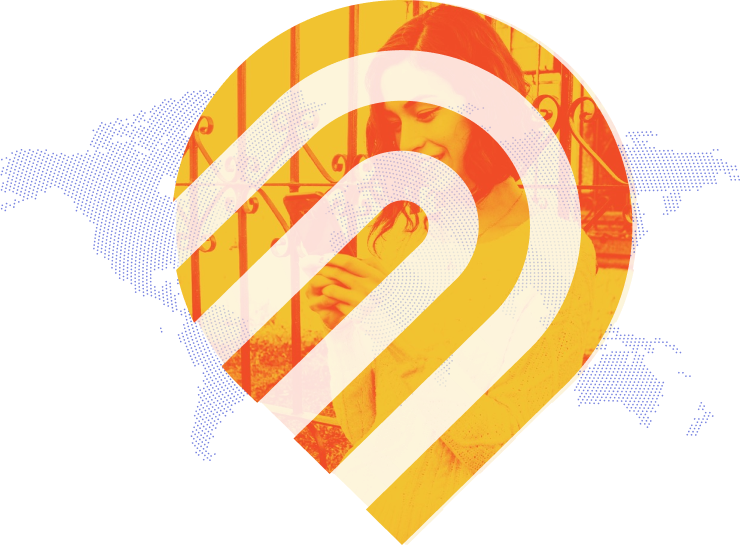
Discover Unparalleled Global Coverage
0 +
Countries & Territories
0 K+
Brands
0 M+
Locations
0 B+
Observations
The dataplor Difference
Boost your business strategy with data-driven insights fueled by the highest-quality data.
Diverse Data Offerings: Tailored to Achieve Your Business Goals
Global Places Data
Create powerful business strategies with global places data to add geospatial context to your business objectives. Gain valuable insights into global commercial and retail locations with our comprehensive POI datasets, to develop a thorough understanding of your target area allowing you to make business decisions confidently.
Global Foot Traffic Data
Uncover actionable insights with global foot traffic and mobility data from dataplor. Understand the number of visits to a specific location or area and make informed decisions to optimize your business strategy. Our global mobility data provides valuable information on traffic patterns and traffic trends so you can better understand consumer behavior, empowering you to tailor your business initiatives to meet the evolving needs of your customers.
Location Intelligence Data
Enhance understanding of your area of interest and create business strategies that have a greater impact to thrive in the rapidly evolving environment. dataplor’s location intelligence data bolsters analyses of customer preferences and local trends, enabling you to tailor your business approach to meet those demands effectively.
Proprietary Metadata
Access insights you won’t find anywhere else, such as confidence scores, place attributes, and consumer preferences that are difficult to uncover without the right tools. Leverage this data to identify emerging trends and capitalize on them.
Geometry Data
Visualize how customers interact with the physical environment they inhabit and uncover hidden patterns with dataplor’s geometry data. This data is offered for all POIs, to provide critical information on location performance. The geometry data can be used to combine global places data to identify expansion opportunities or potential customer segments.
Brand Data
Identify strategic investments, analyze competitor performance, and understand consumer behaviors for over 15,000+ brands. dataplor’s brand data gives access to real-time information on a variety of topics, such as brand health metrics, foot traffic and growth activities, with more brands added daily. This comprehensive dataset provides insights into market share, helping you make informed decisions on where to invest resources.
Investing in Excellence: Our Extensive Quality Control Techniques for Superior Data Quality
Data is vital for any organization, and generating accurate insights is crucial for reliable analytics. Supercharge your tech and business strategy with top-notch location intelligence, using AI-driven data extraction and human verification to fuel global growth.
Human Validation
dataplor excels in human validation through a powerful combination of AI-driven automation. Unlike our contemporaries (or other providers), we don’t rely on academics to validate data. We hire a highly trained in-house team of local experts who bring:
- Never outsourced data QC to academics; only employ in-house regional data experts
- Fluent proficiency in local languages for accurate assessment
- Higher level reasoning to resolve complex data discrepancies
- Continuous training and enhancement of AI models, driven by careful capture and utilization of manual human interventions
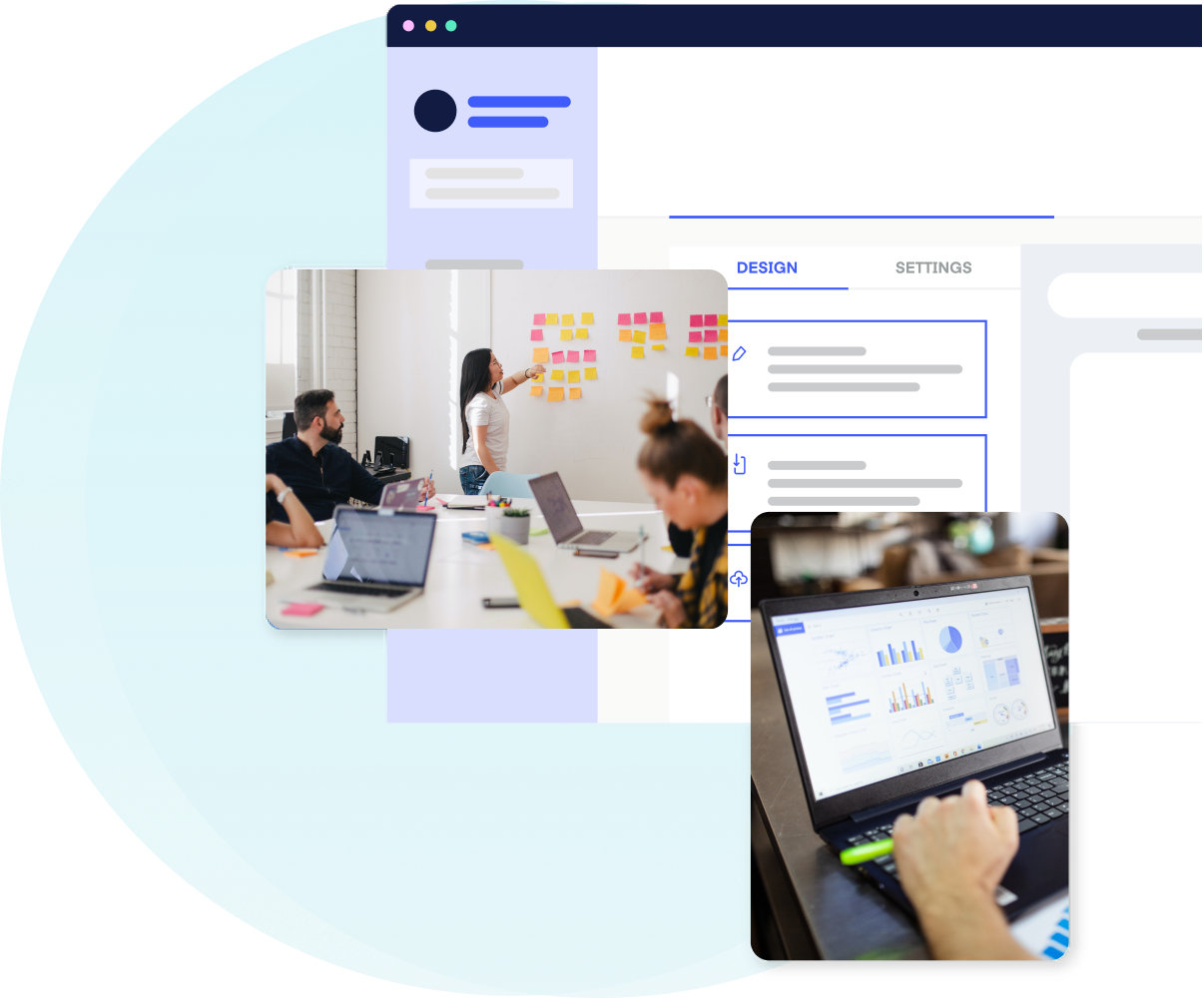
Machine Learning
Experience the power of dataplor’s advanced machine learning capabilities, designed to bring you unparalleled geospatial insights and accuracy through:
- Rapid and accurate pattern recognition
- Real-time extraction of useful information from vast geospatial datasets
- Efficient processing of billions of data points, while identifying potential errors and assigning confidence score
- Comprehensive accountability system for data accuracy
- Ingesting of data from thousands of sources at near real-time
- Breaks down information into individual facts for a unified, single truth record
- Consolidation of data from various sources into a comprehensive, singular record
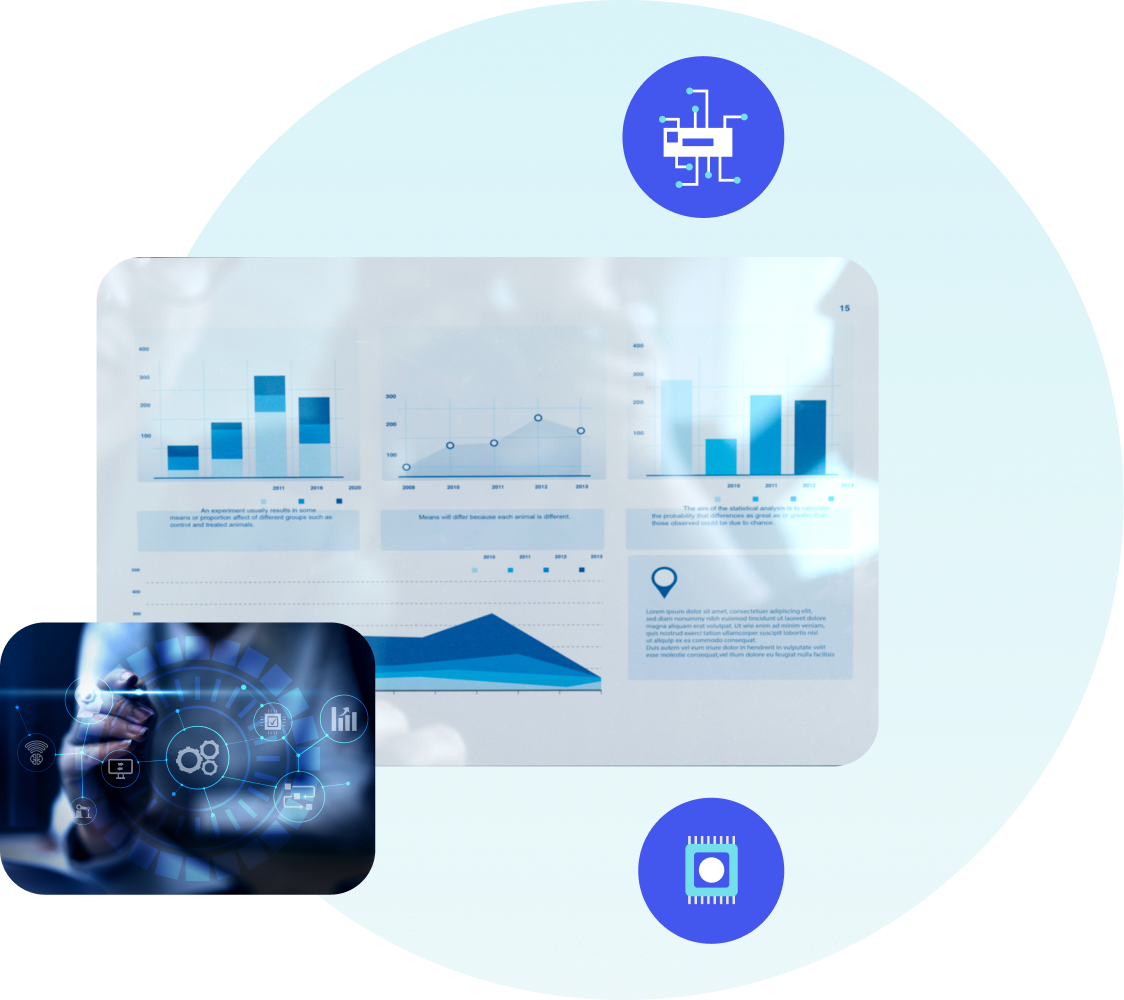
Deep Learning Image Recognition
dataplor’s advanced image recognition technology provides global intelligence on any brand, any place in the world. Enabling you to:
- Understand brand performance across markets over time
- Identify investment and growth opportunities
- Analyze customer preferences and visualize brand presence
- Discover brand associations with specific points of interest
- Gain insights into spatial brand awareness
- Uncover gaps in sales and marketing strategies
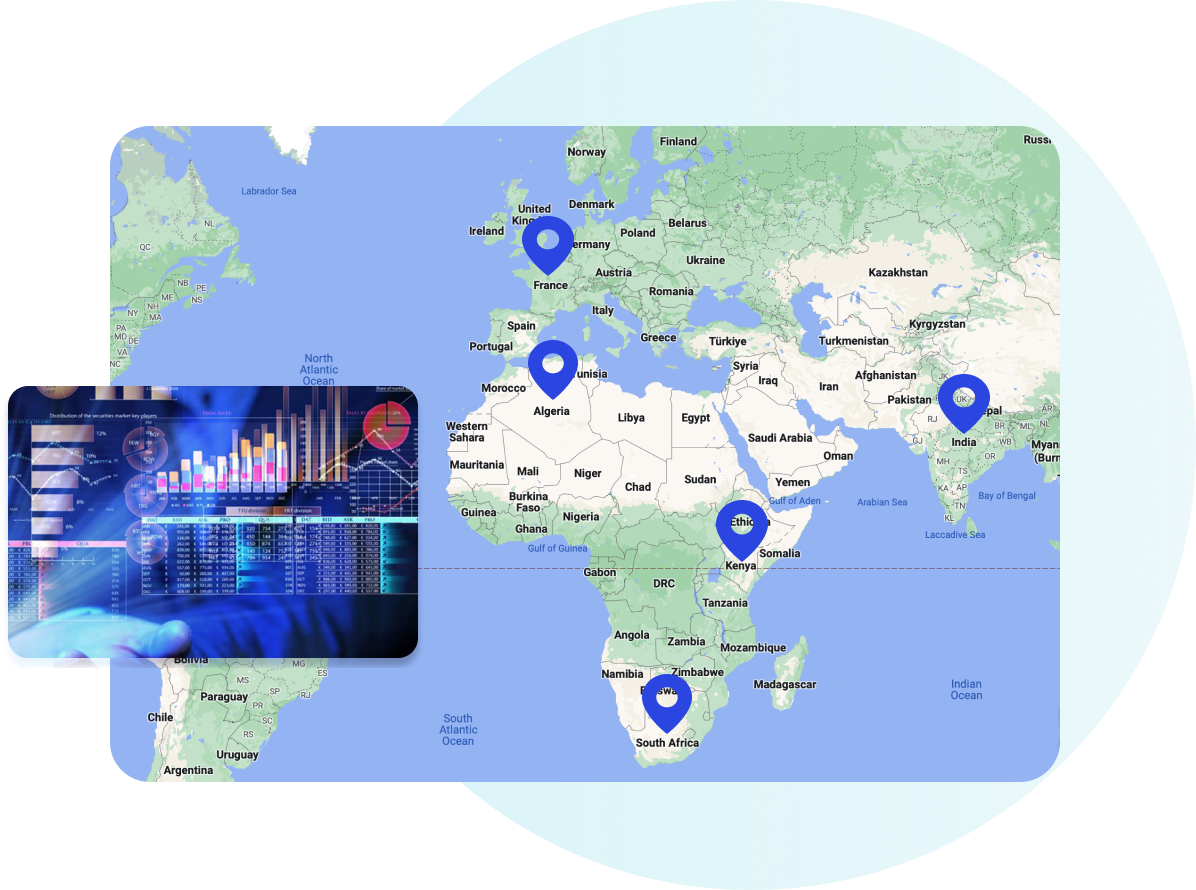
AI Call Bots
dataplor’s AI Call Bots, verifiy crucial information about businesses, to ensure accurate data and enhance overall reliability.
- Fast, automated, AI-driven conversations
- Engage directly with local places in their language
- Guarantee accurate data and verify crucial business information
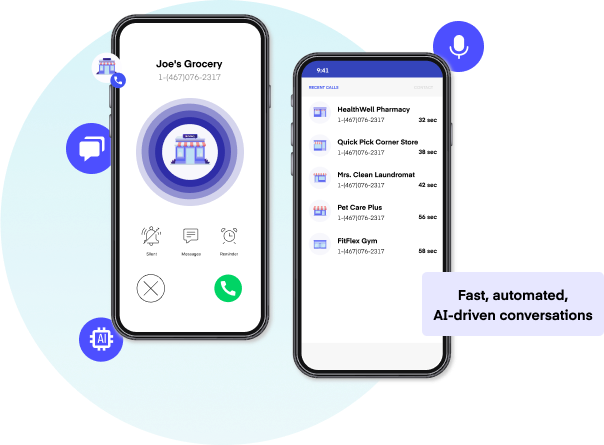
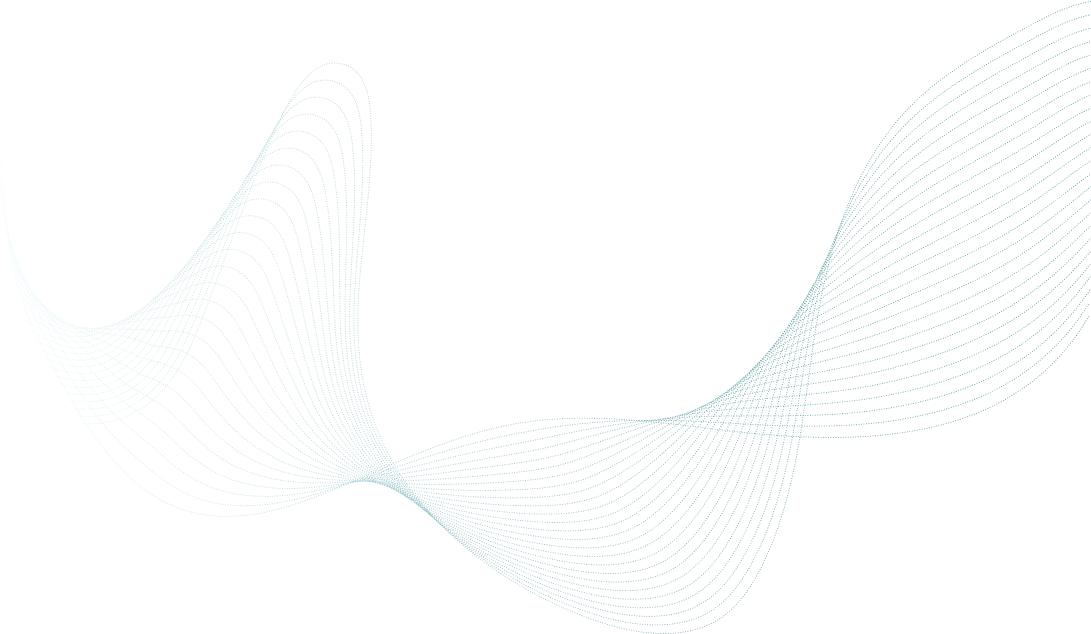
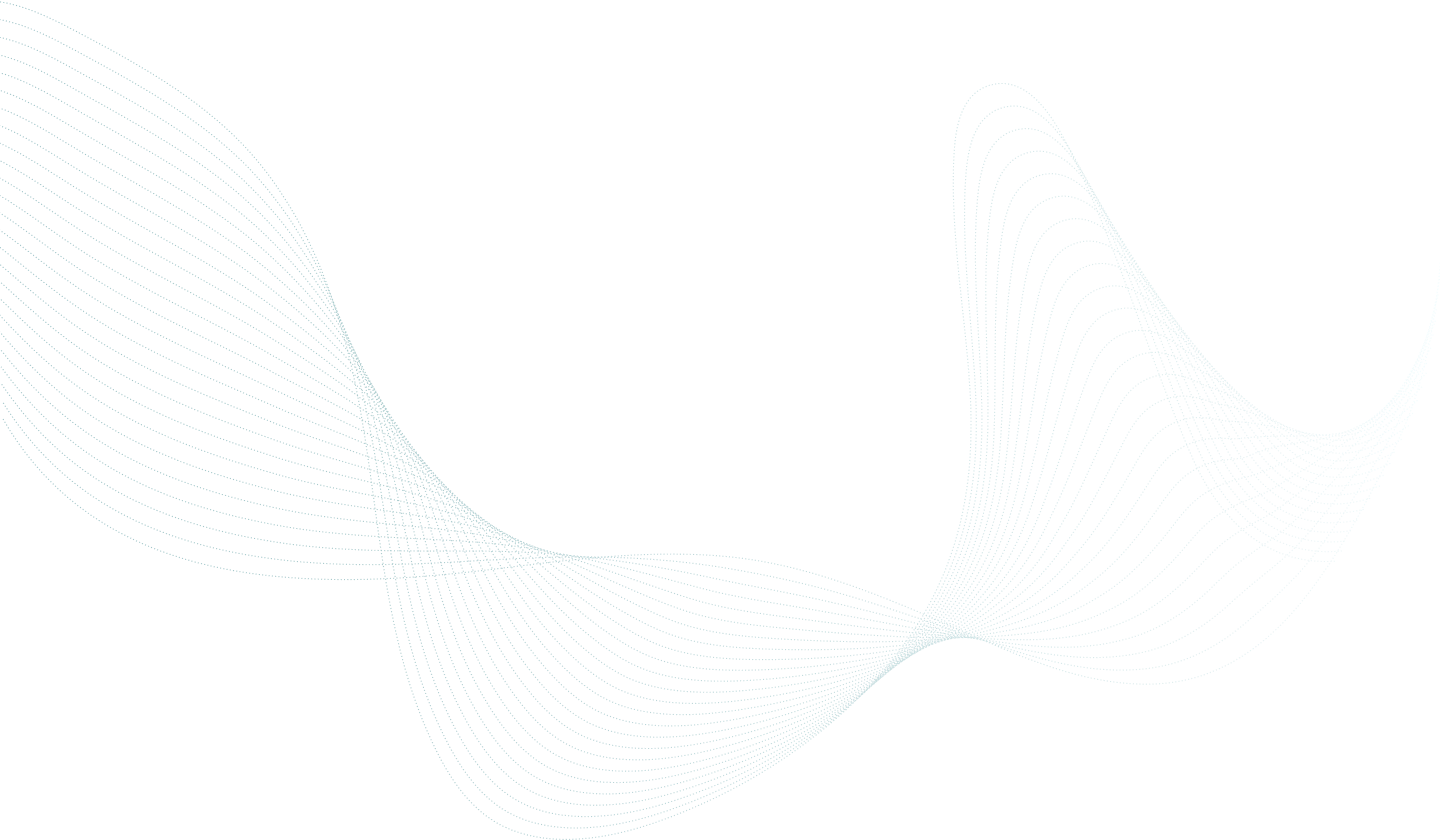
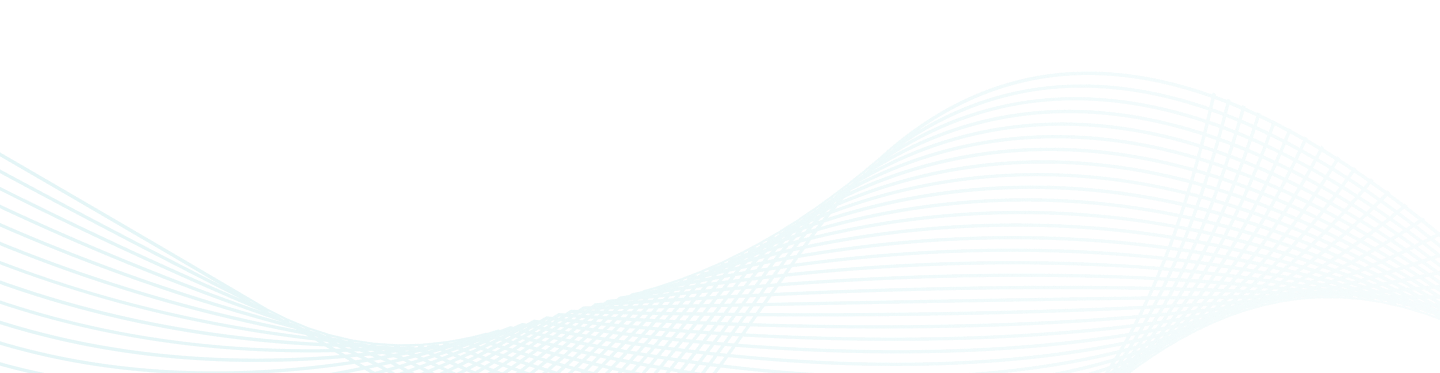
Frequently Asked Questions
How often is the data updated?
Our data is updated in near real-time to ensure you have access to the latest information.
What regions do you cover?
Our POI data covers all regions worldwide, providing comprehensive data across even the most data-scarce or remote regions. Our foot traffic data is currently countrywide across the United States, Mexico, and Canada.
How is the data reviewed?
We subject our data to a thorough review process involving AI, ML, and human scrutiny to guarantee the utmost accuracy and reliability. This meticulous procedure encompasses manual inspections and data verification conducted by our team of skilled experts.
Can I scale this data globally?
Absolutely! All our POI data is standardized globally, allowing seamless integration across various markets and regions. Plus, our mobility data currently spans the entire US, Mexico, and Canada.
How do I get started?
Just click the “Contact Us” button in the top right corner of your screen, submit your region of interest, and one of our experts will get back to you within minutes.
How do you package your data?
We offer data on a country-by-country basis, providing comprehensive POI location data for over 250 countries and territories and visitation data across the United States, Mexico, and Canada.
Does your data adhere to privacy laws?
Yes, our data adheres to stringent privacy laws like GDPR. No PII (Personally Identifiable Information) is collected or used, ensuring our POI and mobility data go beyond traditional practices by safeguarding user privacy.